WARNING: Long rant. Shield your eyes and read with a fluffy animal, narcotics, and a bucket for vomit/stool within reach.
Great! A meta-analysis on smartphone apps for depression.
UPDATE: After I wrote this blog, Joe Firth got in touch with me on Twitter. He explained that (paraphrasing here) the reason for rating studies as “low” risk of bias was that if the target variable of interest (depression in this case) was reported in the paper, it shouldn’t really matter whether it was or wasn’t included in the trial registration. Although I see merit in that argument, I would still be distrustful of any study that has funny things going on between the trial registration and publication of the final study. Personally I would prefer to see all studies without a verified prospective trial registration rated as being at “unknown risk of bias”, since we simply cannot know what’s missing and what isn’t. For example, researchers might have chosen to measure both the CES-D and PHQ-9, but left out the CES-D scores because the results were non-significant – without a prospective trial registration, we’d have nothing to go on.
The Mental Elf is a website which usually provides excellent, readable blogs by researchers who look at new studies, critically examining them and presenting annotated results to a broad audience in accessible language. Do visit them and give them a follow on Twitter.
Recently they featured a blog on a 2017 meta-analysis by Firth et al, published in the prestigious journal World Psychiatry (link to fulltext). This being my field of study, I was of course very happy to see a meta-analysis on this subject, especially in such an authoritative journal.
I was excited to see a meta-analysis on this subject, and the Mental Elf blog was convincing: but being an inveterate methodological terrorist and an incorrigible pedant, I checked out the Firth meta-analysis. It was preregistered in PROSPERO (albeit just 2 days before searches were performed), but it is still a good thing and going beyond most meta-analyses in the field.
Thus having high expectations, but also knowing the dire state of research in the field, my heart sank when I saw the risk of bias assessment for the included trials. Although Firth et al. claim to have followed Cochrane’s guidelines for assessing risk of bias (see Handbook), it was immediately clear that they hadn’t really, at least not how I would have.
Before my rant starts, I’d like to stress here that I’m not singling out Firth et al because I hate their meta-analysis, or because they have a publication in a prestigious journal and I don’t, or because they looked at me in a funny way at a conference – I’ve seen excellent earlier work from the authors and John Torous is one of my favourite authors in the field of mHealth.
However, their meta-analysis is a good example of the lack of real assessment of bias in most meta-analyses. It’s an expectation thing: I expect meta-analysts to go beyond a perfunctory “we scanned the paper and everything seemed to be in order” to provide an in-depth synthesis of the available evidence, and to annotate on the generalisability of findings. Maybe my standards are simply too high, but as soon as I started looking into the meta-analysis I started… Seeing things.
Selective^2 outcome reporting?
How did I know about the not really following Cochrane’s handbook? Because of a prolific research practice that is endemic in most research on eHealth and psychological research in general: selective outcome reporting. Or in the case of meta-analyses: selective selective outcome reporting.
Traditionally, meta-analysts check whether study authors actually report all the stuff they said they would be measuring to prevent something naughty called ‘selective outcome reporting’. For example, your Amazing Intervention™ for depression is finished and you set out to measure depression as its primary outcome measure. Moreover, you’re interested in anxiety and stress, because you know that’s related to depression. But! Shock horror, you find that Amazing Intervention™ only has an effect on anxiety. Problem? Well… What if you just report outcomes for anxiety and tell the world that Amazing Intervention™ works wonders for anxiety? Nobody need ever know about the null findings for depression and stress, right?
To prevent this from happening in trials, and randomised controlled trials (RCTs) specifically, a number of trial registries exist (WHO, ISRCTN, ANZTR) where, ideally, researchers state a priori what, how and when they’re going to measure something. That way it is much harder to hide results, or to claim that an intervention was effective for something while in reality the researcher just kept looking until he/she found something that looked good.
Over the years, I’ve become quite suspicious of selective outcome reporting bias summaries in meta-analyses: most of them are demonstrably wrong if you actually bother to check trial registries against published research, like Cochrane advises. I’m currently working on a paper that investigates naughty stuff in trial reporting for eHealth trials in anxiety and depression (see preliminary results on this poster which I presented at a conference in 2017. Yes I know it’s an awful poster, I was in a hurry).
In that project, I compare trial protocols from international trial registries to published reports of those studies – and a consistent finding is that in many studies, outcome measures change, disappear, appear, or are switched between primary and secondary outcome measure between the protocol and the published papers. So where does the Firth meta-analysis come in?
What’s up with the Risk of Bias assessments?
In Firth’s meta-analysis, the red flag is their summary table of their risk of bias assessment (table 2 in the published paper). As you can see: for all studies, the risk of bias assessment for selective outcome reporting is “low risk of bias” (coded as “+” in the table; highlights are mine).
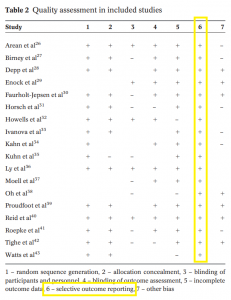
Well, this seemed highly improbable to me.
Firstly, in a sample of 18 studies it is highly unlikely that none of these studies have issues with selective outcome reporting.
Sec0ndly, if you follow Cochrane’s handbook; you’re supposed to trace trial protocols and compare those to the published work (see section 8.14.2 in the Cochrane handbook here). Now, outcome switching or omission is naughty, but it needn’t be an issue for a meta-analysis if the outcome of interest is reported – it’s a moot point whether a primary outcome is reported as secondary, or vice-versa – as long as the outcome is there.
Yes, well, and? Well… 5 out of 18 included studies don’t have a trial registration available, or at least they’re not in the paper and I couldn’t find them in trial registries (cf. Enock et al., Howells et al., Kuhn, et al., Oh et al., Roepke et al.). Most of these were submitted to journals that claim adherence to ICMJE guidelines, which has required prospective preregistration of trials since 2005 (source). Most of the papers themselves claim adherence to the Helsinki declaration which, as not many people know, has required public and prospective preregistration since the update in 2008 (source, see points 21, 22, 35, 36). Nobody seems to check for this.
My opinion: In all of these 5 cases, the risk of bias assessment should read “unknown” since we simply cannot know whether selective outcome reporting took place.
Moreover, Arean et al’s paper points to a trial registration which is very obviously not the same study (Clinicaltrials.gov NCT00540865, see here). That protocol refers to a different intervention (not an app in sight) in a different population, and specifies recruitment times which don’t even overlap with the paper. At first I thought it might be a simple typo in the registration number, but the Arean authors repeatedly and consistently refer to this registration in several other papers on this particular app. Quite an editorial/reviewer oversight in multiple places. Again, this should’ve read “unknown” risk of bias at best, and perhaps “high” risk of bias.
Moreover, the study from Ly et al. has PHQ-9 and BDI as prespecified primary outcome measure (see Clinicaltrials.gov protocol). The published study has the PHQ-9 relegated to a secondary outcome measure, but for this meta-analysis that’s not an issue. However, Firth et al report using only the BDI in Table 1 rather than pooling the effect sizes of PHQ-9 and BDI, as they say they would in the methods: “For studies which used more than one measure of depression, a mean total change was calculated by pooling outcomes from each measure.” (Firth et al., 2017, p288). This is odd, and might be an oversight, but it is a deviation from both the methods section and the PROSPERO protocol.
Missing, presumed accounted for.
Missing data is the scourge of eHealth and mHealth research, and this is no different in the Firth meta-analysis. Even though all but 2 included studies score a “low risk of bias” score for incomplete outcome data (see figure 2 in Firth), it is questionable if any type of missing data correction can account for substantial amounts of missing data.
For example, look at figure 1 in Arean et al., which shows post-test attritions of 26-33%, going up to 65-77% at follow-up. Is this an issue? Well… This is where applicaton of the Cochrane handbook comes down to interpretation. Here’s what they have to say about missing data:
“The risk of bias arising from incomplete outcome data depends on several factors, including the amount and distribution across intervention groups, the reasons for outcomes being missing, the likely difference in outcome between participants with and without data, what study authors have done to address the problem in their reported analyses, and the clinical context.” (source; emphasis mine).
It comes down to interpretation: were the missing data approaches from study authors ‘good enough’ to account for systematic differences in missing data between different arms in the RCTs? The answer could be “yes”, resulting in “low risk of bias” for the “incomplete outcome data” criterion.
Then again, it could equally be argued that large amounts of missing data – endemic in eHealth/mHealth studies – are something that cannot simply be fixed by any kind of statistical correction, especially since these corrections almost always assume that data is missing at random, which they seldom are (for an excellent discussion about these issues by people way cleverer than me, see Mavridis et al here). Either way, you’re left with an imprecise result, which is not the meta-analysts’ fault – but it is something that should be discussed in detail rather than dismissed as “accounted for” and checked off with a “+” in a risk of bias table.
Clearly, this is an area where the Cochrane handbook could use some updating, and the interpretation of whether missing data are ‘accounted for’ is a judgement call. However, relying on study authors to address missing data, in my opinion at least, misses a major point of a meta-analysis: to give an objective-as-possible synthesis of available evidence and to catalogue and discuss its limitations in relation to the generalisability of the results; which is something that – with respect to all authors – I find lacking in both the Mental Elf blog and the Firth meta-analysis.
Wait, there’s more.
- Two included studies have an obvious financial conflict of interest: Arean et al. and Roepke et al., both of which include authors paid by the app developer (see here and here[paywalled], respectively). This is usually coded as “other sources of bias”, and as such Firth et al. have coded this correctly in Table 2. Or at least I assume that’s the reason for coding these studies as high risk of bias – there may be more reasons. But none of this is discussed in the paper, which may represent an author choice – I think it would have been good to include this essential information since a) both studies report some of the highest effect sizes in the meta-analysis, and b) neither of these studies have a study protocol available, leaving the door wide open for all sorts of questionable research practices and methodological ‘tricks’. I’ve reviewed a number of mHealth papers recently: quite a few were methodologically weak high-N ‘experimercials’ conducted by mobile app firms. These had no trial registrations available (at best, retrospective ones).

- There is something very odd going on in the main forest plot (figure 2 in the paper), which for some reason not adequately explained or explored mixes studies with active and wait-list control conditions. This was registered in the protocol, but to me this makes no sense, why would you pool the outcomes of such differing interventions with different control conditions? (this is probably where a large part of the statistical heterogeneity comes from – see table 3 in Firth).
- The pooled g=0.383 from this forest plot is also the ‘main’ finding for the paper. Again, this makes no sense. Much less so when you read carefully and realise that these figures are – granted, as per protocol – changes in depressive scores, i.e. pre-posttest effect sizes. There are quite a number of problems with this: for starters, pre-post measurements are not independent of each other, and calculating effect sizes this way needs the pre-post correlation which is usually unknown (For a more comprehensive explanation of why you shouldn’t use pre-post scores in meta-analyses, see Cuijpers et al here). Secondly, despite randomisation, differences in pre-test scores can still exist. Randomisation is not some magical process that makes differences at baseline disappear.
- Finally, the inclusion of studies using completers-only analyses means that the pre-post scores will be skewed towards the ‘good’ patients that bothered to fill in post-test measurements (see also “missing data”).
- Also, the column headings for “Lower limit” and “Upper limit” appear to be switched in figure 3. This is probably Comprehensive Meta-analysis’ fault, which is a horrible buggy piece of software).
- The reference to Reid et al, 2014 is obviously incorrect: that paper only reports secondary outcomes from the trial. The correct reference should be Reid et al, 2011. Not a biggie, but it’s a bit sloppy.
“No evidence of publication bias”?
Hahahaha! No but seriously. Of course there is publication bias, it’s a given. It’s just that we’re probably not able to detect it very well. Here’s a screenshot from the WHO trials register, where I did a quick dirty search for ((smartphone OR mobile) AND (depression OR mood)) up until Jan 1st 2016 (I generously don’t expect anything after that to be published yet).
Notice anything? It’s the “Results available” column. Some of these studies go back a decade. Many of these studies were never published. Of course there is publication bias, and this is only the preregistered studies – the Firth et al. sample alone contains 5 unregistered studies, Zarquon knows how many unregistered ones are out there (this is what makes publication bias an ‘unknowable unknown‘).
The tests currently available for checking publication bias are synthetic, statistical measures which are highly dependent on the data. At best, they are mostly useless, at worst, they are positively misleading: especially when they ‘conclude’ that there is no evidence of publication bias, as in Firth et al’s case. For an interesting overview of current methods and their performance in meta-analyses, see Carter et al here.
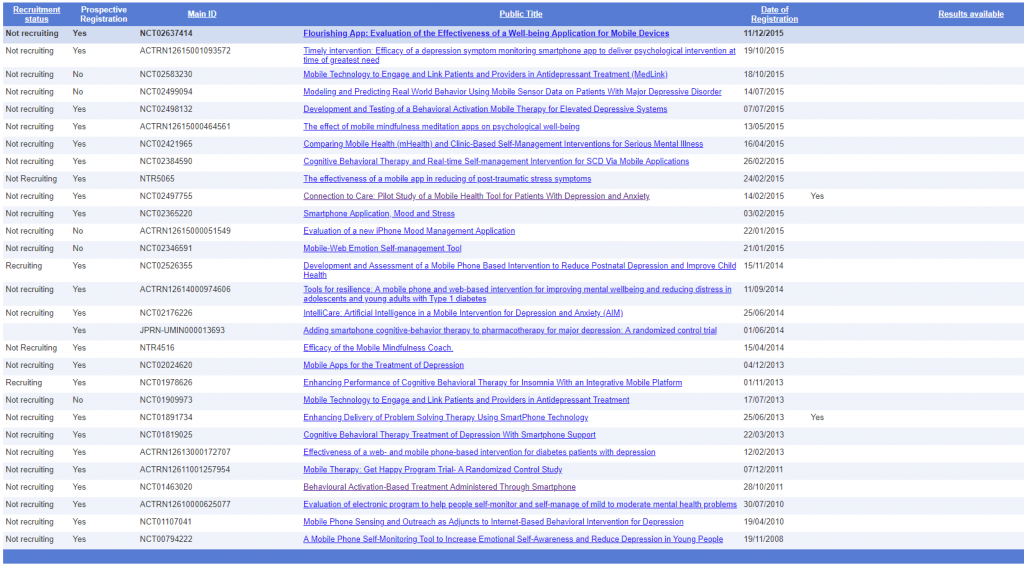
Frail Fail-safe….No!
Apart from other ways to detect publication bias, Firth et al report that “Additionally, a “fail-safe N” was used to account for the file draw[sic] problem” (p.289); and happily report that “…the fail-safe N was 567 (estimating that 567 unpublished “null” studies would need to exist for the actual p value to exceed 0.05).” (p.292).
Please, meta-analysts, for the love of Zarquon, it’s 2018, stop using Fail-safe N. Only people who want to desperately “prove” something the efficacy of something use that. Perhaps we should abandon tests for publication bias altogether since it’s an “unknowable unknown”, and reports of “no publication bias” are profoundly misleading.
Are you quite done yelling, Dr Kok?
OK, now what? Well… Probably nothing. I’m probably wrong about all of this, nobody is going to care, nothing is going to change, this blog will be read by 2 people and a Google bot, and that’s it. Because who am I to argue?
In the end, it’s mostly disappointment on my part. Again, I’ve seen earlier work from the authors of this meta-analysis and generally it’s been very good. The field needs a good meta-analysis on mHealth. I’m confident the Firth and team had the best intentions of the world, they pre-registered the meta-analysis in PROSPERO, the results are available as an Open Access paper and yet… I somehow expected a more in-depth discussion from a meta-analysis in a prestigious journal (if you’re the deluded type that thinks the IF says something about quality (it doesn’t), World Psychiatry has staggering impact factor of 26.561, but using the IF is for the statistically illiterate, so… Erm let’s not go there now).
Firth et al. will probably be widely cited as an authoritative meta-analysis; especially since the take-home message of this meta-analysis for a lot of people will be “apps are moderately effective in treating depression”. But the field of mHealth deserves a more nuanced discussion of all the caveats and methodological issues that restrict the generalisability of findings in quite substantial ways.
So I’m actually hoping that I’m wrong about all of this rather than that the authors dropped the ball on a number of things. Oh, the same author team has published another meta-analysis on apps looking at anxiety – I did not look at this study in detail but it shares some of the same studies included in this meta-analysis on depression apps. Find that meta-analysis here (paywalled unfortunately).
What’s the take-away?
Well, for my part it reinforces a point made before by others: the quality of research into mHealth is very, very dubious, so much so that we cannot draw any reliable conclusions on the efficacy and effectiveness of mHealth interventions for depression. My fear is that the Firth et al. meta-analysis will cause an oversimplification effect: future trials for mHealth application will duly power their analyses to detect an expected effect size of g=0.4 without knowing exactly where this effect size came from.
TAKE HOME MESSAGE: Triallists, meta-analysts, colleagues: our field deserves better. We can do better. We should do better.
Final note
The blog authors on Mental Elf conclude by repeating a cheap shot at the RCT I’ve been hearing a lot in the past years: the RCT is apparently ‘too slow’ to keep up with technological developments. There is nothing inherently “slow” in the randomised controlled trial, but ineffective and poorly piloted recruitment practices in eHealth trials have given led to disappointing accrual rates of participants, which in turn have given the RCT an undeserved bad rap (after all, it couldn’t possibly be the case that researchers have wildly overestimated their ability to attract participants, right?).
A bad workman blames his tools, so don’t blame ineptitude in recruiting participants on the RCT. If eHealth and mHealth are to be evidence-based, they are to adhere to rigorous standards of scientific research. So don’t ditch the RCT just yet, do proper pilot studies, be realistic and optimise your recruitment strategies rather than blaming the RCT as a research tool.
Reference:
Firth J, Torous J, Nicholas J, Carney R, Pratap A, Rosenbaum S, et al. The efficacy of smartphone-based mental health interventions for depressive symptoms: a meta-analysis of randomized controlled trials. World Psychiatry. 2017;16(3):287–298. PMID: 28941113 [Open Access]
CONFLICT OF INTEREST STATEMENT:
Throughout the digging through studies and writing this blog I was repeatedly conflicted, and interested in cracking open a beer and blasting some Motörhead instead of wasting my spare Friday ranting on the Internet. I opted for rooibos tea and Lana del Rey instead.
This blog is CC BY-NC. Feel free to tell me how wrong I am on Twitter.
One thought on “About Firth’s “Apps for Depression” meta-analysis…”